Machine Learning is Transforming the Healthcare Industry, Says Philip Nelson, Engineering Director at Google by CDT
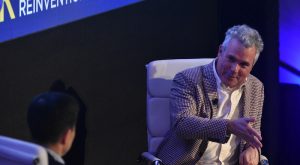
Today, 10 percent of the world has diabetes, and about a third of diabetics deal with diabetic retinopathy. It’s a slow-moving degenerative disease, and the fastest growing cause of blindness.
But new technologies offer hope for increasing diagnostic accuracy and potentially improving care, according to Philip Nelson, director of engineering at Google Accelerated Science. Nelson spoke at the Center for Digital Transformation’s Road to Reinvention Conference in March.
Technology is opening up new possibilities in healthcare, especially in the developing world. Greater medical expertise is becoming available to understaffed parts of the world, such as India and China. House visits, not from doctors, but from clinical workers with machines able to detect a medical crisis and send patients to the nearest clinic, are becoming a possibility.
Google has gathered enough images of the retina from patients at risk for retinopathy to feed those images into a neural network and train a machine to detect the red and orange spots that indicate the disease. In turn, this has allowed Google to improve diagnostic accuracy from 80 percent to more than 90 percent.
This development hinges on the study of the retina fundus –– the only place in the body where you can visualize vasculature and neurons, which provides a tremendous window into what’s going on in the body.
“Just by looking at your retina, we can also determine your cardiac risk, blood pressure, if you smoke, and even your born gender,” Nelson said. “Machines are quite accurate, and they’re helping us discover things that we never knew before.”
Of course, machine learning comes with its complications, too.
For instance, you could mount a camera over a billiard table, and the moment the cue hits a ball, you would be able to learn where the ball is going. But you wouldn’t be able to understand the physics behind it –– the laws of friction or the elasticity of bumpers.
In other words, machines are great at making observations, but not so great at communicating underlying mechanisms, and perhaps most important, determining how scientists and engineers can act on the data.
“Machines are gigantic correlation engines. Correlation doesn’t equal causation, but correlation is highly correlated with causation,” Nelson laughed. “So it’s an exciting time, but there are also ways to use machine learning in the wrong way.”
Today, 10 percent of the world has diabetes, and about a third of diabetics deal with diabetic retinopathy. It’s a slow-moving degenerative disease, and the fastest growing cause of blindness. But new technologies offer hope for increasing diagnostic accuracy and potentially improving care, according to Philip Nelson, director of engineering at Google Accelerated Science. Nelson spoke at the Center for Digital Transformation’s Road to Reinvention Conference in March. Technology is opening up new possibilities in healthcare, especially in the developing world. Greater medical expertise is becoming available to understaffed parts of the world, such as India and China. House visits, not from doctors, but from clinical workers with machines able to detect a medical crisis and send patients to the nearest clinic, are becoming a possibility. Google has gathered enough images of the retina from patients at risk for retinopathy to feed those images into a neural network and train a machine to detect the red and orange spots that indicate the disease. In turn, this has allowed Google to improve diagnostic accuracy from 80 percent to more than 90 percent. This development hinges on the study of the retina fundus –– the only place in the body where you can visualize vasculature and neurons, which provides a tremendous window into what’s going on in the body. “Just by looking at your retina, we can also determine your cardiac risk, blood pressure, if you smoke, and even your born gender,” Nelson said. “Machines are quite accurate, and they’re helping us discover things that we never knew before.” Of course, machine learning comes with its complications, too. For instance, you could mount a camera over a billiard table, and the moment the cue hits a ball, you would be able to learn where the ball is going. But you wouldn’t be able to understand the physics behind it –– the laws of friction or the elasticity of bumpers. In other words, machines are great at making observations, but not so great at communicating underlying mechanisms, and perhaps most important, determining how scientists and engineers can act on the data. “Machines are gigantic correlation engines. Correlation doesn’t equal causation, but correlation is highly correlated with causation,” Nelson laughed. “So it’s an exciting time, but there are also ways to use machine learning in the wrong way.”